What do deepfakes, real-time payments, and grandma buying $5,000 in gift cards have in common? They're all examples of how traditional transaction monitoring is being pushed to its limits.
This isn't just about fraud—it’s about speed, psychology, and systems being tested by an entirely new playbook.
And let’s be honest: most current systems just throw more alerts at the problem.

You can’t solve modern fraud by layering monitors on monitors. Real protection requires systems that understand context—not just trigger alerts. Transaction monitoring needs to be smarter, not just louder. The threats aren’t only more sophisticated—they’re harder to detect, faster to act, and increasingly human-shaped.
In this piece, we’ll break down what scammers are actually doing right now, why legacy monitoring tools aren’t enough, and what it takes to move from static rules to strategic foresight.
Real-World Tactics from the Modern Fraud Playbook
Kyle Thorton, Project Manager here at iDENTIFY and a veteran in fraud and risk operations—with 10 years of experience, including as Director of Fraud Ops and Risk Management (and self-proclaimed former rapscallion)—put it this way: “Scammers have shifted from creating fake accounts to taking over real ones. It’s cleaner. Faster. Easier to hide.”
In this environment, transaction monitoring requires more than just reactive flagging. It requires the ability to think like a criminal. Think “Catch Me If You Can”, where Frank Abagnale Jr., a young hot shot master check forger, evades capture for years before becoming one of the FBI’s top fraud experts. To catch the con, you have to understand the con artist.

Here’s what the landscape looks like today, and what we need to be looking for:
- Deepfaked onboarding:
Fraudsters inject synthetic video into liveness checks using widely available tools. Some biometric systems still can’t tell the difference. In one major case, a group of fraudsters in China used purchased facial images to create live deepfakes and spoofed facial recognition systems, setting up a shell company to issue fake tax invoices totaling over $76 million. All with tools that cost as little as $250. Systems must evolve to validate not just the image, but the authenticity of the data stream. - Behavioral mimicry:
Real users autofill sensitive info. Fraudsters usually paste. Real users know their SSN. Bad actors type it slower or switch tabs. Monitoring systems can watch for behavioral clues—but the next challenge will be differentiating human from bot in increasingly convincing simulations. - Device analytics as insight—not just logging:
Tools should monitor how long a user takes to input known data (like SSN), whether autofill is used, tab switching behavior, and even if the keyboard or mouse behavior appears human. These micro-patterns, when stitched together, reveal intention. The more volume or automation is used by the attacker, the more detectable these become. - Social engineering that overrides logic:
People still buy $5,000 in gift cards to pay the “IRS.” Even when warned by store clerks or bank reps. This isn’t just a transaction problem—it’s a psychological one. Systems need to be flexible enough to intervene based on anomalies and help educate customers at risk. - Real-time payment fraud:
Once funds hit RTP rails or are off-ramped to crypto, they’re gone. The margin for error is milliseconds. This shift demands proactive rather than reactive monitoring—tools that flag patterns rather than waiting for thresholds to be crossed.

The throughline: assume the adversary is already here, and already evolving. Our job isn’t just to catch them—it’s to stay one move ahead.
One major example: real-time payment (RTP) networks. As Kyle pointed out, RTP has created a faster lane not just for legitimate transfers, but for fraud. Because RTP activity happens within the same-day network and the actual external account movement doesn’t settle until later, there's a dangerous illusion of finality—when in fact, there’s a gap in oversight.
In the past, scammers stole accounts and opened new ones to move funds. Now, with many consumers owning multiple fintech accounts, a fraudster can compromise several under the same identity and make transfers that appear normal at first glance. Many systems still don't verify name-to-name consistency on transfers, and in the U.S., unlike in Europe, mismatched names aren’t always flagged. That creates blind spots.
And once funds are off-ramped to crypto? They're effectively untraceable. Fraud has moved to where speed is highest and verification is weakest. That’s why transaction monitoring must evolve—not just in speed, but in sophistication.
More reading about deepfake:
-> Contextualizing Deepfake Threats to Organizations
Beyond the Alert: Designing for Real Human Behavior
Zelle and the Price of No Diligence
Zelle offers a real-world cautionary tale. As Kyle explained, when major banks rolled out the peer-to-peer product, it entered the market with almost no oversight. The risks weren’t unknown—platforms like Cash App had already experienced similar issues—but the assumption seemed to be: "we’re different." That assumption proved costly. According to the Consumer Financial Protection Bureau, consumers reported more than $870 million in fraud and scam-related transfers over Zelle in a single year.
Despite being backed by some of the largest financial institutions in the U.S., Zelle’s structure lacked critical fraud prevention protocols. When scams began to surface, users and regulators alike questioned: who’s responsible? The customer who authorized the transfer—or the bank that built a system where fraud was both easy and irreversible?
The takeaway: when financial institutions build fast-moving products without sufficient guardrails, they inherit the risk. And when fraud happens, the public expects accountability—not excuses.
As compliance professionals, we’re trained to trust evidence, rules, and process. But what happens when fraud mimics normal behavior almost perfectly? When identity is spoofed but behavior looks typical?
We’re entering a new era. One that asks us to weigh freedom and personal responsibility, with the ethics of security and safety.
Freedom vs. Security
Perfect security might look like a chip embedded in your hand or iris scans à la Minority Report—a world where identity is absolute, and transactions require nothing short of biometric validation. But as every science fiction fan knows, that kind of security comes with a cost: centralized control, loss of autonomy, and a slow drift toward surveillance over service (remember that iconic mall scene in Minority Report?)
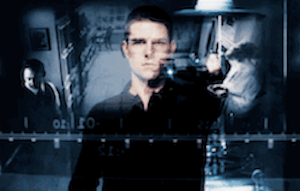
Banks constantly have to weigh
- Friction vs. security
- User experience vs. systemic risk
- Automation vs. human judgment
This isn’t just a technical challenge. It’s an ethical one—and a human one.
Every added layer of defense—whether it’s a device check, behavioral scan, or transaction delay—creates friction. But reducing friction too far creates exposure.
And here’s the hard part: the weakest point in nearly every system is still people. As Kyle said, the fact that passwords still exist in 2025 is a problem in itself. People reuse them. Share them. Click links they shouldn’t. Fall for social engineering. Even SMS-based two-factor authentication (2FA) is vulnerable to SIM swaps.
What’s needed, he argues, is a cultural shift toward mandatory multi-factor authentication (MFA)—and not the clunky kind. Seamless, secure authentication needs to be the default across all financial access points. Until then, we’ll continue patching systems that are only as strong as their least informed user.
So how do we balance both?
Start by clarifying intent:
- Are your controls designed to catch every anomaly—or to catch the right anomalies?
- Does your team have the power to override automation when human context matters?
- Can your tools adapt rules based on what you learn in real time?
A great example of this in practice: a bank compliance team notices an uptick in a new type of fraudulent transaction pattern—perhaps money being transferred in a specific sequence across fintech apps. With modern tools, they should be able to instantly create a new rule at the bank level and push that rule down across every fintech partner they support. No waiting on each fintech’s internal compliance analyst to get to it. No fragmented response times. Just centralized visibility and action. That kind of agility isn’t just operationally efficient—it’s critical in a world where fraud evolves daily.

Ethical monitoring is about proportionality. It’s about designing systems that intervene where the risk warrants it—and back off where it doesn’t. But it also raises a deeper question: who holds the responsibility for preventing harm?
When 80% of users follow clear signs and behave safely, what do we do about the 20% who ignore the warning and walk straight toward a cliff? If the institution knows the cliff is there—and that some will miss the sign—do they put up a fence? Post a guard? Redirect traffic entirely?

In banking, this is more than a metaphor. Customers fall victim to scams even after being warned. And regulators increasingly expect that institutions do more than just "show the sign." They expect you to protect people from falling.
Balancing customer autonomy with institutional duty isn’t easy. But in the end, if a user is manipulated into authorizing a fraudulent wire, the bank is still the last line of defense. Ethical monitoring is not just about data and logic—it’s about care. And as discussed in the context of peer-to-peer payment risks by Banking Dive, the expectations for institutional diligence are rising, regardless of who 'initiated' the transaction. It’s about asking: what’s our obligation to protect people, even from themselves?
The Current Landscape of Transaction Monitoring Tools
Today’s fraud detection isn’t just about rules—it’s about context, counterparty, and behavior. Here’s a breakdown of key platforms shaping the space:
Platform: Hawk AI
Known For: Counterparty screening + real-time analytics
Ideal Use Case: Banks managing multiple fintech programs or BaaS partners
Platform: Unit21
Known For: No-code rule engine with customizable thresholds
Ideal Use Case: Fast-changing environments, especially sponsor banks needing agility
Platform: Sardine
Known For: Advanced device analytics (e.g. emulator detection, copy/paste tracking)
Ideal Use Case: Onboarding and session-level fraud detection for digital-first institutions
Platform: Oscilar
Known For: AI-powered risk decisioning with real-time behavioral models
Ideal Use Case: Forward-leaning compliance teams exploring predictive risk tools
Platform: Verafin
Known For: Traditional AML coverage and legacy fraud scenarios
Ideal Use Case: Banks focused on regulatory reporting and long-established fraud detection
Each tool solves different parts of the fraud problem. But none of them are magic. According to recent analysis in Fintech Brainfood, what separates effective systems from the rest isn't just speed—it's interpretability, and the ability to make judgment calls across shifting behavioral norms. As fraud tactics become more complex, detection systems must go beyond alerts—and toward interpretation.
Final Thoughts: We Can Catch You and We Will
Fraud today moves faster than most institutions are built to handle. It's more psychological than procedural—and increasingly human-shaped. Tools alone aren’t enough. We need mindset shifts.
To stay ahead, banks must think like the very adversaries they’re trying to outmaneuver. That means spotting intent in behavior, not just anomalies in numbers. It means designing systems that adapt quickly, balance freedom with protection, and prioritize people without sacrificing accountability.
Because at the end of the day, institutions aren’t just protecting money—they’re protecting people. And when people walk toward the metaphorical cliff, the public expects banks to do more than warn them. They expect us to stop them.
So how do we move forward?
- Think like a criminal.
- Protect people, not just processes.
- Design for real behavior, not perfect users.
That’s how you stay one move ahead.